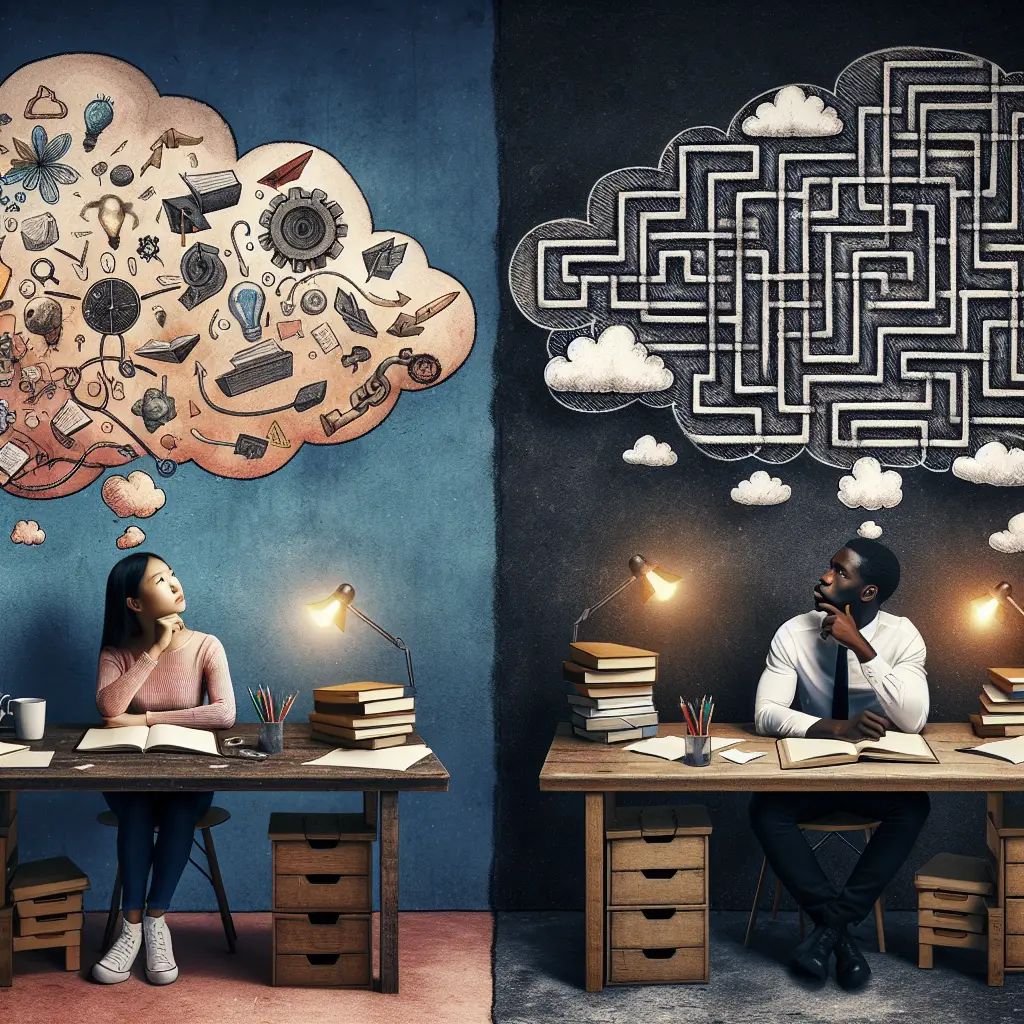
In the realm of problem-solving, the emergence of artificial intelligence (AI) has introduced novel methodologies that promise to revolutionize how we approach complex issues. Among these innovative methods, Chain of Thought Prompting and Traditional Problem Solving stand out as two dominant approaches. This comparative analysis delves into their functionalities, advantages, and the recent advancements shaping their evolution.
Understanding the Core Concepts
Chain of Thought Prompting is a cutting-edge technique primarily used in AI problem solving. It involves structuring prompts to guide AI in a step-by-step reasoning process, emulating human cognitive strategies to reach a conclusion. This method has been pivotal in enhancing the decision-making process within AI systems, making them more transparent and aligned with human reasoning.
Traditional Problem Solving, on the other hand, is a more established approach used by humans. It encompasses various problem-solving models and techniques that rely heavily on experience, intuition, and structured methodologies like the scientific method or the PDCA (Plan-Do-Check-Act) cycle. This approach is deeply rooted in cognitive psychology and is essential for tackling issues where a direct logical structure is absent.
Comparative Analysis: Efficiency and Cognitive Load
The efficiency in problem solving varies significantly between these two methods. Chain of Thought Prompting leverages AI's ability to process vast amounts of information rapidly, often leading to quicker solutions in data-intensive scenarios. For instance, OpenAI’s Project Strawberry showcases how AI can perform 'Deep Research' to reason and generate solutions based on extensive data sets [Source: OpenAI]. Conversely, Traditional Problem Solving might be slower but allows for deeper human insight and ethical considerations, essential in scenarios where data doesn't tell the whole story.
Cognitive load in problem solving also differs greatly. AI Problem Solving, particularly with techniques like Chain of Thought Prompting, often reduces the cognitive load on humans by handling complex computations internally. However, it requires careful prompt engineering to ensure alignment with desired outcomes, as seen in OpenAI's recent advancements in prompt engineering strategies [Source: OpenAI]. Traditional methods, while potentially more cognitively demanding, foster a comprehensive understanding of the problem space through hands-on engagement.
Recent Innovations and Their Implications
Several recent developments highlight the evolving landscape of problem-solving techniques:
Embodied Reasoning in Robots: Researchers have developed techniques enabling robots to exhibit 'embodied reasoning', integrating physical interactions with cognitive processes, thus enhancing AI problem solving capabilities in real-world scenarios [Source: MIT News].
ChatGPT as a Data Analyst: Initiatives to use ChatGPT for data analysis are underway, illustrating AI's potential to streamline complex data interpretation tasks [Source: TechCrunch].
Radar Trends to Watch: The August 2024 radar trends indicate a growing emphasis on AI prompting techniques and their integration into mainstream applications, signaling a shift towards more sophisticated AI problem-solving tools [Source: Gartner].
Rephrase and Respond Strategies: This innovative prompting strategy has significantly improved AI's ability to understand and respond to complex prompts accurately, marking a significant step forward in AI-human interaction [Source: AI Journal].
Non-Obvious Prompt Engineering Guide: A recent guide offers insights into unconventional prompt engineering techniques that could redefine how problems are approached in AI settings [Source: DeepMind Blog].
These innovations not only demonstrate the rapid advancements in AI problem-solving but also highlight the increasing synergy between human cognitive strategies and artificial intelligence.
Human vs AI Problem Solving
The debate between Human vs AI Problem Solving often centers around the balance of efficiency, ethics, and empathy. AI systems, equipped with capabilities like those seen in Project Strawberry from OpenAI, are becoming adept at handling complex research and decision-making tasks independently. Yet, they lack the inherently human ability to integrate ethical reasoning and emotional understanding fully into the decision-making process, an area where Traditional Problem Solving excels.
The Future Trajectory
As we look towards the future, it's evident that both Chain of Thought Prompting and Traditional Problem Solving will continue to evolve. The integration of advanced cognitive strategies within AI systems suggests a trend towards more nuanced and sophisticated problem-solving methods. Tools like computational thinking are becoming indispensable in leveraging AI effectively within problem-solving contexts [Source: Harvard Business Review].
Conclusion
In conclusion, both Chain of Thought Prompting and Traditional Problem Solving have their unique strengths and limitations. The ongoing developments in AI Problem Solving are promising but require careful consideration of ethical implications and human values to ensure technology remains a beneficial tool for humanity.
As we continue to explore these fascinating realms of human intellect and machine capabilities, it becomes increasingly clear that the fusion of human insight with artificial intelligence holds unmatched potential for solving the myriad challenges facing our world today.
By Henry Clarkson